Meta’s Five New AI Tools: Making Machines a Little Less Robotic
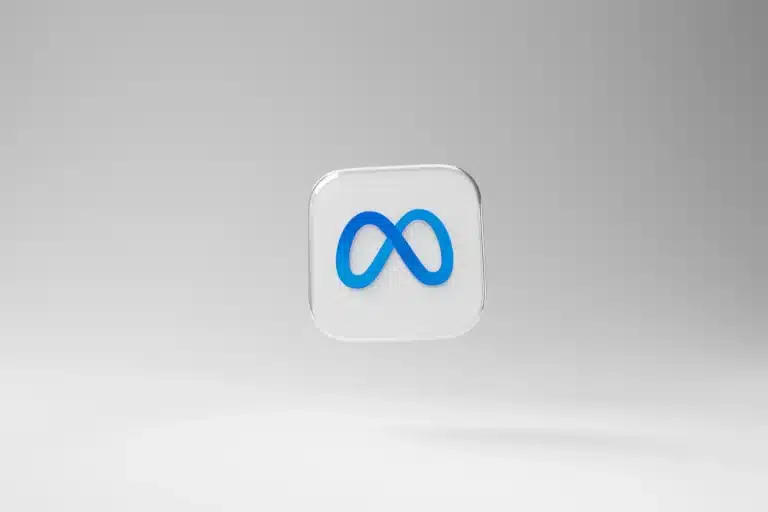
Meta’s Fundamental AI Research (FAIR) team just dropped five major releases designed to make AI a lot more like us—minus the coffee addiction and existential dread. These projects target the core challenge of advanced machine intelligence: getting machines to actually perceive, reason, and interact with the world like humans do, not just crunch numbers in a server farm.
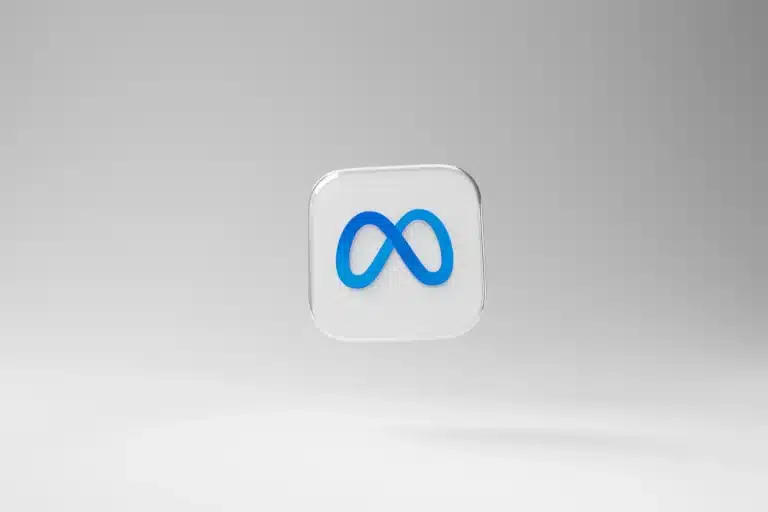
Photo by Dima Solomin on Unsplash
The Big Five: Meta’s Latest AI Breakthroughs
1. Perception Encoder: Giving AI Actual “Eyes”
Most AI vision models can spot a cat in a photo, but ask them to find a stingray buried in sand or a goldfinch hiding in the background, and they’ll probably just shrug (if they had shoulders). Meta’s Perception Encoder is a large-scale vision model that excels at zero-shot classification and retrieval—meaning it can identify things it’s never seen before, in both images and videos. It’s robust, bridges vision and language, and even stands up to adversarial attacks. When paired with language models, it boosts performance on tough tasks like visual question answering and understanding spatial relationships (think: “Is the mug behind the laptop?”)125.
2. Perception Language Model (PLM): Open-Source Vision-Language Power
PLM is Meta’s answer to the “black box” problem in AI. It’s a fully open, reproducible model trained on a massive blend of synthetic and human-labeled data—no proprietary shortcuts. PLM isn’t just about recognizing objects; it’s about understanding complex visual scenes and linking them to language. Meta is also releasing a new benchmark, PLM-VideoBench, to test fine-grained activity recognition and spatiotemporal reasoning, areas where existing benchmarks fall short. The goal? Give researchers the tools to build and test truly transparent, high-performing vision-language systems (1)(5).
3. Meta Locate 3D: Robots That Know Where Stuff Is
If you’ve ever yelled at a robot vacuum for getting stuck under the couch, this one’s for you. Meta Locate 3D lets robots find objects in a 3D environment using natural language queries (“Find the flower vase near the TV console”). It processes 3D point clouds from depth sensors and links them to language, so robots can understand not just what you want, but where it is in the real world. Meta’s also doubling the available annotated data for this task, helping robots get a lot less lost—and a lot more helpful (1)(8).
4. Dynamic Byte Latent Transformer: Language Models That Don’t Choke on Typos
Traditional language models break text into tokens, which is great until someone types “teh” instead of “the.” Meta’s new model skips tokenization and works directly with raw bytes, making it more resilient to misspellings, rare words, and adversarial inputs. The 8-billion parameter model is not only efficient, but also outperforms token-based models in robustness tests. Meta’s releasing the weights, so the research community can see if this byte-based approach is the future of language AI (1)(5).
5. Collaborative Reasoner: AI That Can Actually Work With You
AI isn’t known for its social skills. Meta’s Collaborative Reasoner is a framework for training and evaluating AI agents that can collaborate—think helping with homework, prepping for interviews, or brainstorming solutions. It tests for multi-step reasoning, communication, empathy, and the ability to disagree constructively. Meta uses synthetic data where AI agents collaborate with themselves, improving performance on complex tasks by up to 29.4% compared to solo models. The pipeline is open-sourced to encourage more research into truly “social” AI (1)(5).
Why This All Matters
These aren’t just five random projects—they’re interconnected pillars supporting Meta’s vision of Advanced Machine Intelligence (AMI). The goal? Machines that don’t just compute, but perceive, reason, and interact like humans. From sharper vision to smarter collaboration, this is the infrastructure for the next generation of AI that’s not just smart, but intuitive and adaptable.
Meta’s approach is also refreshingly open. By releasing models, datasets, and benchmarks, they’re inviting the entire research community to build on this foundation—accelerating progress for everyone, not just the big players.
The Takeaway
If you’re waiting for the moment when AI finally “gets it”—when it can see, understand, and work with us like a real partner—Meta’s latest FAIR releases are a giant leap in that direction. Whether you’re a researcher, a developer, or just an AI enthusiast, this is the stuff you’ll want to keep your eyes on.